Many state-of-the-art approaches to extract information from big Earth observation (EO) data have their foundation in statistical “black box” methods, which are trained on increasingly large datasets. Such approaches have proven significant success for specific applications, documented by performance criteria such as accuracy and speed.
However, we see two critical inherent shortcomings of today’s approaches: The lack of semantics (being able to create and maintain the human-interpretable meaning of EO image contents) and explainability (allowing humans to comprehend how a result was produced). The first shortcoming refers to the system’s inability to have an inherent understanding of the concepts that are being processed. While being able to identify objects, systems do not know their meaning. The lack of semantics limits in EO analyses in three aspects:
- The expressiveness of inferences (create new information by reasoning)
- Transferability and generalization (concepts with varying characteristics such as city, forest)
- Interoperability (connecting with other datasets, e.g., in a knowledge graph)
The second shortcoming refers to the ability to investigate the system’s internal decisions and conclusions about how a result was inferred.
We consider semantics and explainability interwoven and highlight the necessity to solve both simultaneously: Semantics as a pre-condition for enabling explainability and explainability, in turn, as a necessity for interfaces to semantic analyses. In this special session, we invite contributions to semantic analysis and explainability in the big EO domain by investigating them individually or synergistically.
Examples include but are not limited to:
- Semantic enrichment and automated semantic feature extraction
- Intelligent and efficient management of data and information
- Semantic analysis and reasoning
- Workflows for semantic querying
- EO-based knowledge graphs
- Explainable inferences on big EO datasets
- Efforts to create standards and best-practice examples
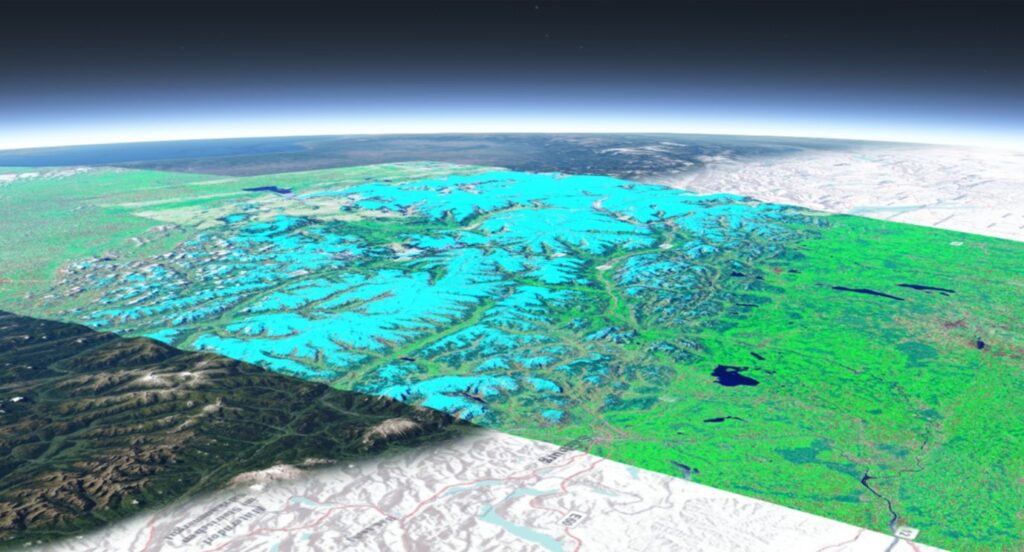
List of topics
- Semantic analysis
- Semantic querying
- Big earth data
- Knowledge graphs
- Intelligent data management
- Explainable artificial intelligence
Organisers
Martin Sudmanns – Department of Geoinformatics, University of Salzburg – martin.sudmanns@plus.ac.at
Dirk Tiede – Department of Geoinformatics, University of Salzburg – dirk.tiede@plus.ac.at.
Hannah Augustin – Department of Geoinformatics, University of Salzburg – hannah.augustin@plus.ac.at
Gregory Giuliani – University of Geneva & UNEP/GRID-Geneva, Switzerland – gregory.giuliani@unepgrid.ch