In recent years, the field of remote sensing has experienced a surge in Earth Observation (EO) data thanks to advances in satellite technology and the launch of innovative constellations. These new satellite constellations have revolutionized data acquisition by enabling the capture of imagery with unprecedented spatial, temporal, and spectral resolutions. This abundance of multi-resolution and multi-modal satellite data, which includes Synthetic Aperture Radar (SAR) and multispectral imagery, has opened new avenues for comprehending the dynamics of our planet.
Deep learning has gained considerable traction in remote sensing, showing significant potential in the analysis of multi-temporal and multi-modal satellite time series. Nevertheless, its widespread adoption is impeded by the challenge of acquiring reliable training labels and developing models capable of generalizing across diverse geographical regions.
Leveraging self-supervised techniques, which reduce the reliance on labelled data for model training, offers a promising solution for extracting valuable insights from Earth observation big data. This session aims to unite researchers, practitioners, and experts in an exploration of the latest advancements, challenges, and applications in self-supervised learning, specifically tailored for remote sensing and Earth observation big data. Together, we aim to address the growing demand for reliable solutions in this rapidly evolving field.
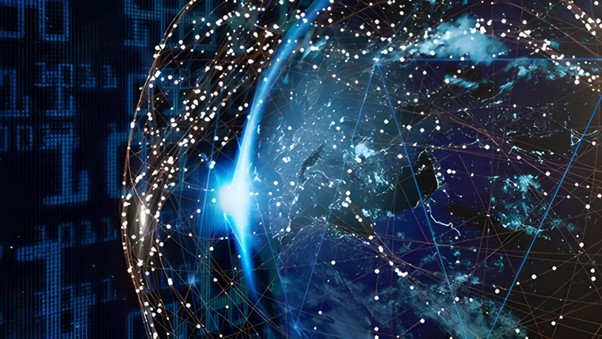
List of topics
The session invites contributions on a wide range of topics related to self-supervised techniques for remote sensing data, including but not limited to:
- Self-supervised pretraining of models for SAR and multispectral data
- Multi-modal self-supervised learning using SAR and optical data
- Representation learning for remote sensing data
- Cross-modal self-supervised techniques for fusion of SAR and multispectral data
- Self-supervised anomaly detection and change detection in Earth observation data
- Self-supervised techniques for geospatial feature extraction
- Transfer learning and domain adaptation using self-supervised methods
- Applications of self-supervised learning in environmental monitoring, disaster management, agriculture, urban planning, and more
- Evaluation metrics and benchmarks for self-supervised remote sensing models
- Combining self-supervised and weakly supervised approaches for enhanced performance
Organisers
Andrea Nascetti, University of Liège, Belgium & KTH Royal Institute of Technology, Sweden andrea.nascetti@uliege.be
Mattia Crespi, University of Rome “La Sapienza”, Italy mattia.crespi@uniroma1.it
Link to the SIG webpage https://rrs.earsel.org/